Could MIT 45 be the key to unlocking a deeper understanding of a complex scientific phenomenon? The term, encompassing a set of sophisticated computational methods and experimental protocols, has, over the past decade, quietly revolutionized fields ranging from theoretical physics to materials science, offering unparalleled precision and insight into previously intractable problems. The very nature of its influence, though largely confined to the research community, speaks volumes about its potential to reshape our understanding of the world around us.
The genesis of MIT 45, while shrouded in the specifics of proprietary research conducted within the hallowed halls of the Massachusetts Institute of Technology, reveals a story of persistent innovation. Driven by the need for more precise, efficient, and reliable methods to study complex systems, a team of researchers began to develop a suite of computational tools, often coupled with cutting-edge experimental techniques. Their goal? To overcome the limitations of existing approaches and push the boundaries of what was scientifically possible. The resulting methodologies and algorithms, optimized through years of refinement, have since become indispensable to numerous research groups worldwide. The initial focus may have been narrow, addressing specific challenges in quantum mechanics. But the impact of the approach quickly broadened. It began to be adopted and adapted in fields such as condensed matter physics, where it was used to model the behavior of novel materials, and in chemical engineering, to design more efficient catalytic processes. MIT 45 wasn't just a scientific breakthrough; it was a paradigm shift. It represented a move towards more sophisticated, data-driven approaches to problem-solving. It empowered researchers with the tools needed to tackle previously impossible computational and experimental challenges.
The core of MIT 45 lies in its multifaceted approach, carefully integrating advanced computational modeling with meticulous experimental design. The computational component often involves sophisticated algorithms and highly optimized software to simulate complex physical and chemical processes. These simulations are typically run on high-performance computing clusters, allowing researchers to model systems with a degree of accuracy and detail that was impossible only a few years ago. This computational work is usually coupled with carefully designed experiments, where data is collected to validate and refine the models. The feedback loop between the computational and experimental components is critical. Results from simulations inform the design of experiments, and experimental data is used to improve the accuracy and reliability of the computational models. This iterative process enables researchers to gradually refine their understanding of the system being studied and make increasingly accurate predictions.
A crucial element of MIT 45 is its ability to handle large and complex datasets. The scale of both computational models and experimental data can be overwhelming. The researchers who developed the method were also instrumental in the development of associated data analysis techniques. These techniques allow researchers to manage, analyze, and visualize vast amounts of information. Sophisticated statistical methods, machine learning algorithms, and advanced data visualization tools are used to extract meaningful insights from the data. This capacity is especially important in areas such as materials science, where the properties of a material are influenced by many factors. MIT 45 allows scientists to isolate the effects of individual factors and to identify the relationships between them. This ability is crucial to the design and discovery of new materials with tailored properties.
The specific methodologies employed within the framework of MIT 45 are, by their very nature, highly technical and tailored to the field of application. While the details will vary from project to project, its possible to identify common threads. For instance, researchers in condensed matter physics might use density functional theory (DFT) calculations to model the electronic structure of materials. This involves solving the Schrdinger equation to determine the behavior of electrons within a solid. The results of these calculations are then used to predict the material's properties, such as its conductivity, magnetism, and optical properties. The computational models are validated against experimental data obtained from techniques such as X-ray diffraction, which provides information about the atomic structure of the material, and scanning tunneling microscopy (STM), which allows researchers to visualize the materials surface at the atomic level.
In the domain of chemical engineering, MIT 45 approaches frequently involve the simulation of chemical reactions. Researchers often use computational fluid dynamics (CFD) to model the flow of fluids and the mixing of reactants. They then apply quantum chemical calculations to study the reaction mechanism, determining the energy barriers for different reactions. This combined approach allows them to design more efficient catalytic processes or to optimize the operating conditions of chemical reactors. This work is often validated against experimental data collected from pilot plants or industrial-scale reactors. The use of detailed computational models, coupled with experimental data, is crucial for process optimization.
One of the most compelling aspects of MIT 45 is its capacity to accelerate the pace of discovery. By providing researchers with the tools needed to make predictions and test hypotheses rapidly, the method shortens the time it takes to identify and characterize new materials, to design new processes, or to understand fundamental scientific phenomena. In essence, MIT 45 is not just a set of techniques; its a catalyst for innovation.
The impact of MIT 45 extends far beyond the walls of the academic institutions that pioneered its development. Companies in a wide range of industries are actively adopting these methodologies to improve their products and processes. In the pharmaceutical industry, MIT 45 is used to design and optimize drug molecules and to predict the efficacy of new drugs. In the semiconductor industry, it is used to model the behavior of electronic devices and to optimize their performance. In the energy sector, it is used to design more efficient solar cells and batteries. The use of these advanced computational and experimental methods is transforming the way industries conduct research and development, leading to more efficient, sustainable, and cost-effective solutions.
The evolution of MIT 45 is a story of ongoing refinement and adaptation. Researchers are constantly working to improve the accuracy, efficiency, and versatility of the methods. New algorithms are being developed, and existing ones are being optimized. Machine learning and artificial intelligence techniques are increasingly being integrated into the process to automate data analysis and improve the accuracy of the models. The development of ever-more-powerful computing resources, such as quantum computers, holds the promise of further expanding the capabilities of MIT 45 and allowing researchers to tackle even more complex problems. As computational power grows and the techniques evolve, the impact of MIT 45 will only continue to grow, as well.
The future of MIT 45 seems undeniably bright. The widespread adoption of these methods across diverse scientific disciplines and industrial sectors shows the transformative power of the approach. The ongoing refinement of the techniques and the integration of new technologies promise to push the boundaries of what is possible in scientific discovery and technological innovation. Whether its about designing better materials, developing more efficient processes, or simply expanding our understanding of the universe, MIT 45 is poised to play a crucial role in shaping the future of science.
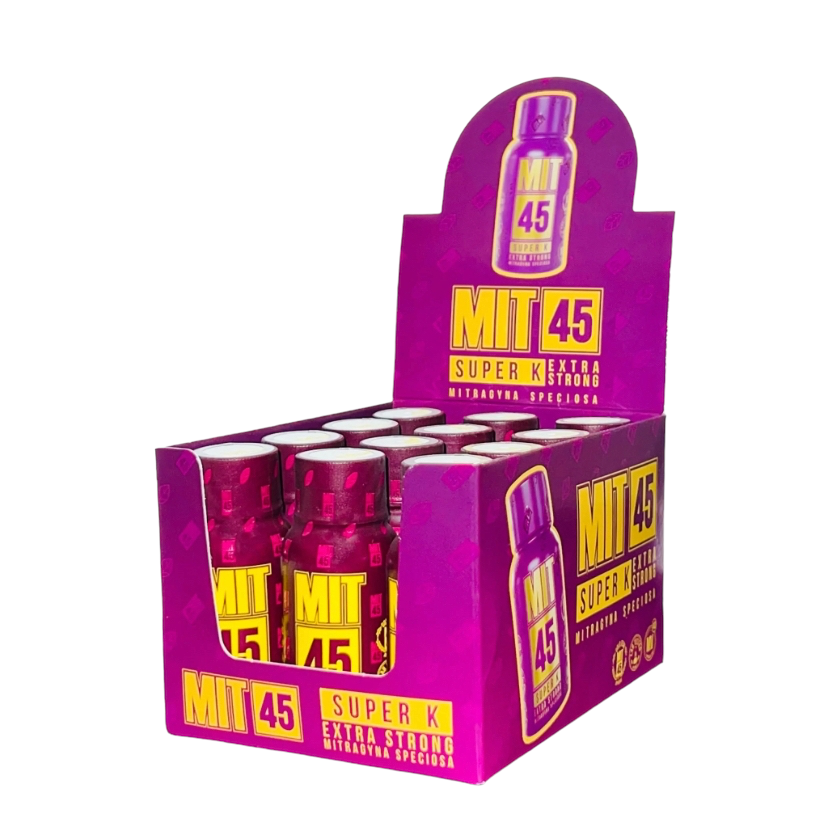

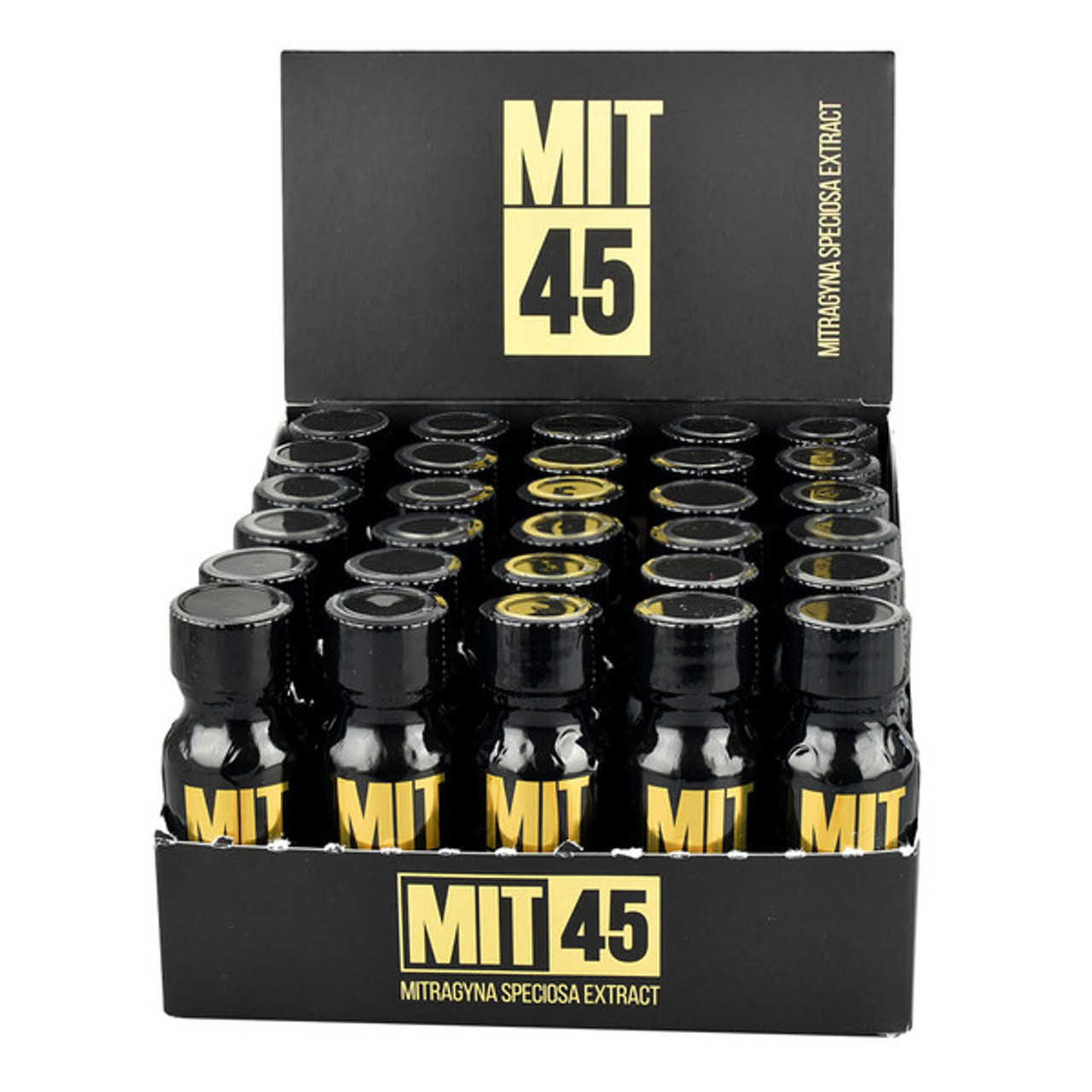